Impact of AI technologies on cardio-metabolic health and drug adherence
- Integrating AI into cardio-metabolic disease management holds promise for enhancing patient outcomes via improved disease detection, risk prediction, and personalized disease management3,5-11.
- Artificial Intelligence (AI) solutions address non-adherence through predictive, assessment, and monitoring tools, transforming patient care13-17.
- Smartphone apps, devices, virtual assistants, education tools, and AI-driven games boost medication adherence, empowering patients and enhancing treatment results3,18-21.
- Challenges include data quality, privacy, ethics, malpractice risks, GenAI hallucinations and implementation complexities4,8.
Navigating the global burden of cardio-metabolic diseases: Is AI the solution?
Cardio-metabolic diseases, encompassing cardiovascular diseases, obesity, and diabetes, are significant contributors to global mortality1. Adhering to medication regimens is noted as crucial for successfully managing cardiovascular disease, as highlighted in our previous article “Medication adherence in cardiovascular disease”. Therefore, integration of Artificial Intelligence (AI) into cardio-metabolic disease management shows promise in improving patient health outcomes. AI, a branch of computer science, aims to extend human intelligence into machines. Machine learning (ML), a subset of AI, utilizes statistical techniques to create intelligent systems2,3. AI offers diverse applications in healthcare, including early detection, diagnosis, and treatment of medical conditions. It also aids in patient engagement, medication adherence, health promotion, counseling, administrative task automation, and professional education within healthcare settings4.
Redefining cardio-metabolic health with artificial intelligence
Before diving into our main topic of addressing medication non-adherence in cardio-metabolic conditions, let’s first explore the impact of AI on cardiometabolic health, as outlined below:
- AI based disease detection and prioritization:
AI-driven algorithms revolutionize electrocardiogram interpretation and cardiac imaging, facilitating rapid and precise diagnoses of cardiovascular conditions5. For instance, neural networks analyzed 91,232 single-lead ECGs, surpassing cardiologists in arrhythmia detection. Utilizing an end-to-end deep learning approach shows potential for accurate classification, potentially reducing misdiagnoses and prioritizing urgent cases6. In cases of ischemic stroke and large vessel occlusions, AI-assisted classification improves disease detection, highlighting the urgent need for swift triage to expedite treatment7,8. Additionally, AI-driven clinical decision support systems (CDSS) provide real-time recommendations based on patient data, identifying drug interactions, formulating low-risk treatment plans, and predicting outcomes, thus enhancing healthcare delivery. Notably, CDSS features such as workflow integration, timely recommendations, and computer-based support significantly enhance clinical practice, achieving an impressive 94% success rate8. - Cardiovascular risk prediction with ML:
Traditional predictive models exhibit limitations such as disparities across validation cohorts, a restricted number of predictors, and the absence of crucial variables5. A large prospective cohort study utilized the AutoPrognosis framework to develop a machine learning (ML) model aimed at predicting cardiovascular events in asymptomatic individuals. Analyzing data from over 400,000 UK Biobank participants, each with 450 variables, study reveal three key insights. First, AutoPrognosis significantly improves cardiovascular disease (CVD) risk prediction accuracy compared to conventional scoring systems like the Framingham score. Second, it identifies new predictors—such as self-reported health ratings and walking pace. Lastly, AutoPrognosis uncovers complex interactions, tailoring CVD risk predictions for specific sub-populations such as individuals with diabetes9. - Multimodal AI approach for blood pressure prediction:
Effective blood pressure control is vital in averting adverse events linked to cardiometabolic diseases like heart attacks, strokes, kidney issues, and mortality. Despite awareness efforts and improved access to monitoring, a concerning 38% of hypertensive adults remain undiagnosed. Only one in four individuals achieve target blood pressure goals, emphasizing the need for better predictive tools. AI-driven research has progressed in defining treatment objectives and predicting medication responses, potentially reducing systolic blood pressure by up to 70%. Integrating biochemical, dietary, genomic, and metabolic data through multimodal AI approaches enhances our understanding of hypertension’s biological origins, paving the way for personalized treatments.
Looking ahead, wearable, cuffless, and continuous blood pressure monitors—incorporating photoplethysmography, ECG, and piezoelectric sensors—offer promise. These bioengineering and machine learning advancements improve accuracy and convenience. Additionally, machine learning analysis of continuous monitors can reveal hemodynamic insights traditionally obtained through invasive methods, enhancing cardiovascular health assessment10. - AI for personalized diabetes management:
A Meta-analysis highlight the promising sensitivity and specificity of machine learning algorithms in predicting hypoglycemia. AI algorithms may provide crucial support in diabetes management, particularly when conventional methods are inadequate. Closed-loop automated insulin delivery systems depend on accurate control algorithms, which analyze continuous glucose monitoring data and adjust insulin infusion rates. These algorithms, including model predictive control, fuzzy logic, and learning algorithms, are essential for closed-loop systems. A wireless closed-loop system integrating fuzzy logic theory and learning algorithms has shown enhanced management of nocturnal hypoglycemia and glucose levels compared to sensor-augmented pumps. Various AI methods have been employed to predict treatment failure in Type 2 diabetes patients on metformin monotherapy, using HbA1c, demographics, and comorbidity data. Additionally, artificial neural networks anticipate therapy escalation, while AI-based tools predict HbA1c changes associated with alternative T2D treatments through an -Electronic health record -integrated system3. - Remote monitoring: AI-enabled wearable devices offer continuous monitoring of vital signs like blood pressure, sleep patterns, stress levels, nutrition, and physical activity, which are key drivers of cardiometabolic diseases. This real-time data enables the early detection of cardio-metabolic issues, facilitating prompt interventions10.
- Behavioral modification and adherence support: AI-driven chatbots and virtual assistants offer behavioral therapy support to help individuals make sustainable lifestyle changes and adhere to weight loss programs11. For more on how AI helps in behavioral modification, please read our article: The role of AI in personalized health coaching for adherence
AI approaching genuine empathy?
In healthcare, empathy, compassion, and trust are crucial values. Healthcare professionals must understand and empathize with patients to provide compassionate care, which positively affects patient satisfaction, treatment adherence, and overall health outcomes. Patients prefer providers who exhibit both skill and emotional intelligence. AI has the potential to improve healthcare by reducing physicians’ workload, enhancing adherence monitoring, and facilitating stronger emotional connections. While AI is not meant to replace bedside physicians, it can greatly enhance patient-physician empathetic communication.
Embracing AI for medication non-adherence: a multifaceted approach
- Predicting adherence: AI and machine learning predict medication adherence, even for complex conditions like diabetes13 and hypertension14. For instance, a smart medication bottle and mobile app collect data on health measures and medication usage. Machine learning algorithms then predict adherence, enhancing patient outcomes15.
- Assessing adherence: AI tools comprehensively assess patient behaviors influencing adherence. Consider an AI-based solution using wireless sensors in patients’ homes. It detects and addresses medication self-administration (MSA) errors during insulin pen use. The system records assessments, uploads data to digital health records, and sends reminders if medication isn’t taken as prescribed. Health professionals access this information via a web portal, enabling targeted interventions16.
- Monitoring adherence: AI may monitor adherence. In a study, an AI smartphone app tracked medication adherence in stroke patients on oral anticoagulants. Using a neural network computer vision algorithm, the app visually identified patients, drugs, and confirmed ingestion. The group with daily AI monitoring achieved 100% adherence, compared to 50% in the control group without daily monitoring— with remarkable 67% improvement17.
Transforming medication adherence in cardio-metabolic conditions using AI:
- Smartphone apps: Utilizing smartphone apps powered by AI, patients benefit from interactive visualizations that enhance medication information retention, with AI-voice technology offering real-time medical status updates for heart failure and diabetic patients18. Telemedicine nurses promptly access Electronic Health Records (EHRs), facilitating faster triage5.
- Smart devices: AI-based smart devices provide real-time monitoring, allowing patients to manage their condition effectively and prevent deviations from prescribed treatment plans. For instance, continuous glucose monitoring systems interpret biomedical data, issuing alerts during hypoglycemic episodes, enabling timely interventions to mitigate associated risks3.
- Virtual assistants: AI-driven virtual assistants play a crucial role in reminding patients to take medications, scheduling appointments, and aiding healthcare professionals in monitoring adherence. In a pilot study involving patients with type 2 diabetes, a significant 76.9% exhibited improved medication adherence with the use of virtual assistants19.
- AI-based education tools: AI-based education tools offer personalized content and advice, fostering patient engagement and enhancing medication adherence. By analyzing electronic health records and home-recorded data, these tools generate visualizations such as HbA1c trends, empowering patients to actively participate in their health management20.
- AI-assisted games: AI-assisted gaming introduces interactive features like challenges and rewards to drive behavior change and patient engagement. Studies have demonstrated reduced calorie intake and enhanced cardiovascular function in younger patients with obesity through AI-facilitated gaming21.
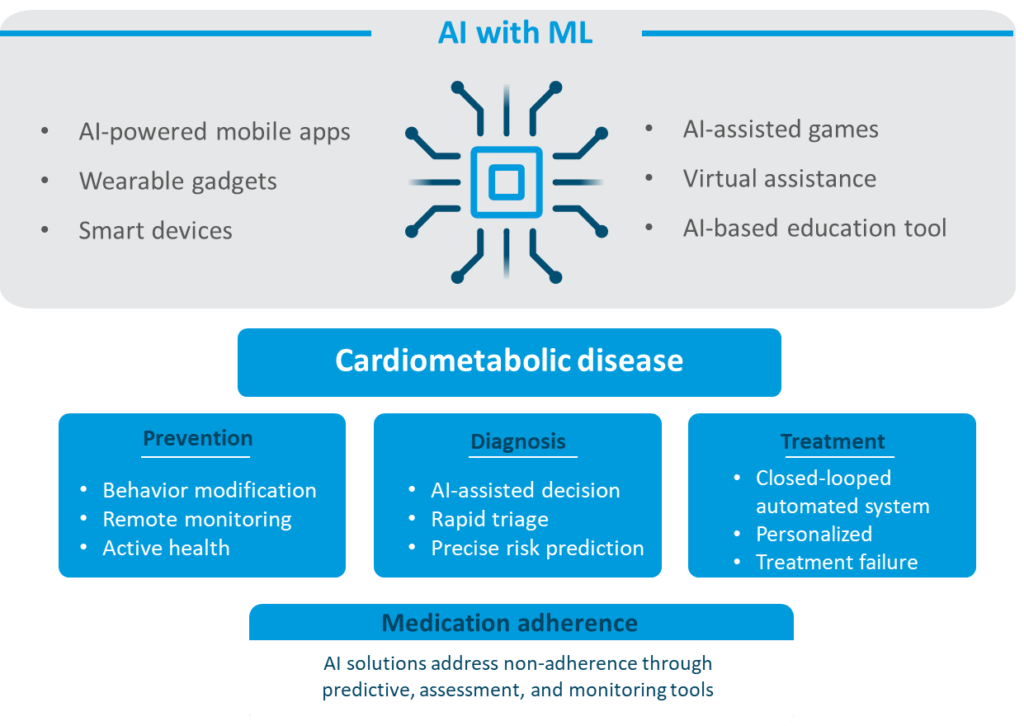
Figure: Artificial intelligence approaches for cardio-metabolic disease management and medication adherence
(Disclaimer: This image was created for illustrative purposes and is based on data obtained from the references: 3,15-17,18-21).
Impact of health app randomized controlled trials (RCTs) on medication adherence and blood pressure
Three RCTs have investigated AI supported health apps for CVD management22. In one study, a smartphone app improved self-reported medication adherence but didn’t significantly impact systolic blood pressure. Another trial evaluated medication reminder apps for coronary heart disease patients, showing self-reported adherence improvements without significant clinical outcomes. Despite limited statistical significance, patients found these apps helpful. A third RCT explored a consumer-directed digital health strategy (CONNECT), which didn’t significantly improve medication adherence but positively affected health behaviors. Physician endorsement and patient-reported enhancements in mental health, physical activity, and diet suggest potential health benefits despite the lack of significant medication adherence improvement.
Table: Recent clinical trials: app-based interventions in CVD patients22
Apps | Interventions | Outcome |
---|---|---|
Medisafe app | Intervention : Medisafe app The Medisafe app offers blood pressure monitoring, tracks medications, provides reminders, generates weekly adherence reports, and includes a Medfriend feature for peer support Control : Usual care | Medication adherence MMAS-8 score—mean (SD) Intervention: 6.3 (1.6) Control: 5.7 (1.8) |
Mobile app | Intervention 1 : Basic app Basic daily reminders similar to alarm or text messages for medication intake Intervention 2 : Advanced app Interactive features allow customization, snooze options, rescheduling, medication tracking, default reminders, refill alerts, adherence stats, data export, and missed dose notifications Control : Usual care | Medication adherence MMAS-8 score mean (95% CI) Intervention: 7.11 (6.90–7.31) Control: 6.63 (6.35–6.92) |
CONNECT platform | Intervention : CONNECT website/app The app integrates with patients’ electronic health records (EHR) for access to personal health data, including medications, test results, blood pressure, and weight. It includes a heart age calculator, basic medication/lifestyle reminders, appointment alerts, motivational messages (e.g., salt reduction tips), interactive goal setting, and peer forums Control : Usual care | Medication adherence %* Intervention: 32.8 Control: 29.9 |
*Medication adherence defined as taking BP and cholesterol medications on ≥ 80% of days
MMAS is a commonly used questionnaire to assess medication adherence in RCTs. It was expanded to the MMAS-8 by adding 4 items to address adherence behavior circumstances, consisting of 7 yes/no questions and 1 Likert-scale rating. Since its inception in 2009, the MMAS-8 has been employed in over 200 studies23.
CI, confidence interval; BP, blood pressure; MMAS-8, Morisky medication adherence scale 8-item; SD, standard deviation
Challenges of using AI:
Despite numerous advantages, adopting AI in cardio-metabolic health faces significant challenges:
- High-quality data for training: AI relies on training data, making data quality crucial. Acquiring unbiased, high-quality data for training and validation remains a challenge4,8.
- Data privacy: AI tools demand robust security to prevent breaches and unauthorized access, especially when handling sensitive patient information4,8.
- Ethical data use: Integrating AI requires ethical scrutiny. Safeguarding patient privacy while training AI systems is essential4,8.
- Generative AI (GenAI) hallucinations: Limitation of GenAI models is extrinsic hallucinations, where they produce unrealistic or nonsensical content inconsistent with the context or data. This poses a risk to areas like software development or critical AI-based solutions, potentially endangering safety, health, or rights24.
- Malpractice: AI’s potential for misdiagnosis or mistreatment raises liability and patient safety concerns4,8.
- Cost & implementation: Initial investments in hardware, software, and staff training strain healthcare budgets. Ongoing maintenance and workflow changes add complexity4,8.
Conclusion
The integration of AI in cardio-metabolic disease management transforms patient outcomes. AI-driven solutions revolutionize disease detection, personalized diabetes management, and medication adherence, utilizing diverse tools like smartphone apps and virtual assistants. Yet, challenges like data quality, privacy, ethics, malpractice, and costs complicate AI adoption. Despite these hurdles, AI holds promise in reshaping healthcare delivery and improving patient outcomes amidst global cardio-metabolic disease burden.
We need to design and build AI that helps healthcare professionals be better at what they do.’” — Mihaela van der Schaar, PhD, Director of the Cambridge Centre for AI in Medicine at the University of Cambridge in the U.K